Databricks is out with its “2024 State of Data + AI Report,” which analyzes the trends in data and artificial intelligence adoption based on the usage patterns of more than 10,000 of their global customers, including 300+ of the Fortune 500.
The central theme is the rapid shift from AI experimentation to production deployment, driven by the emergence of generative AI (GenAI) and the critical role of enterprise data in customizing large language models (LLMs).
Key findings highlight a significant increase in the efficiency of putting ML models into production, the dominance and growth of Natural Language Processing (NLP), the surge in Retrieval Augmented Generation (RAG) using vector databases for LLM customization, the preference for open-source AI tools and models, and the surprising early adoption of GenAI and robust governance practices within highly regulated industries.
The report underscores the importance of “data intelligence platforms” in democratizing data and AI, enabling organizations to accelerate their GenAI projects and realize tangible business value.
Highlights
1.The Rise of Generative AI and the Centrality of Data: GenAI is a transformative force driving innovation, creativity, and productivity, with companies globally investing heavily in its adoption. High-quality GenAI experiences are contingent upon leveraging enterprise data effectively. The urgent question for businesses is how to achieve this rapidly. “Data intelligence platforms,” which utilize GenAI to simplify data security, access and value creation, fostering democratization of data and AI across organizations, will be critical to this process.
2. Transition from AI Experimentation to Production: There is a significant shift underway from merely experimenting with AI to deploying models into real-world applications at scale. The report finds the ratio of logged experimental models to registered (production-ready) models decreased from 16:1 in February 2023 to 5:1 by March 2024, and 11x more AI models were put into production this year. The report attributes the transition, in part, to the availability of data intelligence platforms providing a standardized and open environment for the entire ML lifecycle.
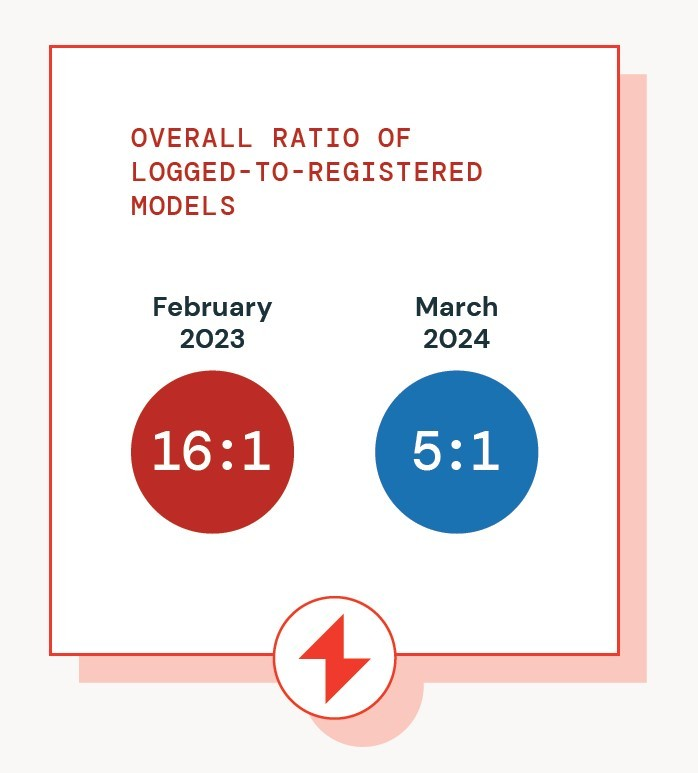
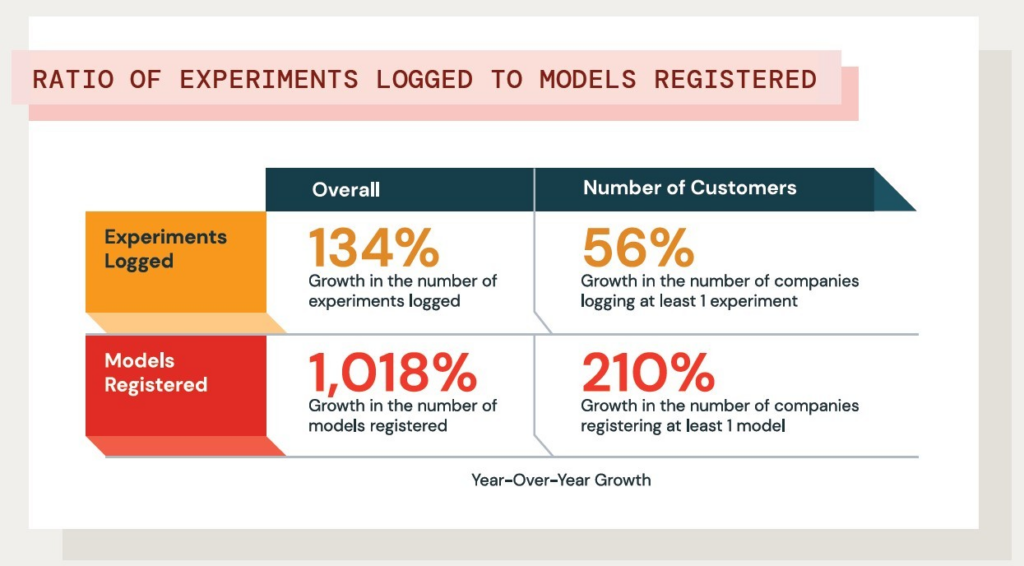
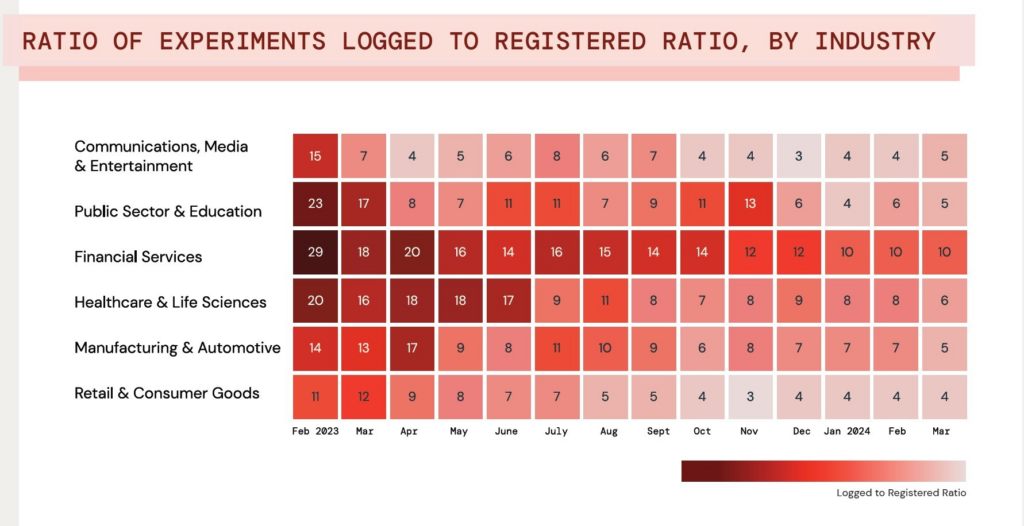
3. Dominance and Growth of Natural Language Processing (NLP): For the second year in a row, the report finds NLP is the most-used and fastest-growing DS/ML (machine learning) application, accounting for 50% of specialized Python library usage. Healthcare & Life Sciences showing the highest adoption (69%). The report highlights significant year-over-year growth in NLP adoption, with Manufacturing & Automotive leading at 148%. This growth is driven by the increasing demand for AI-driven applications that can derive meaning from unstructured data.
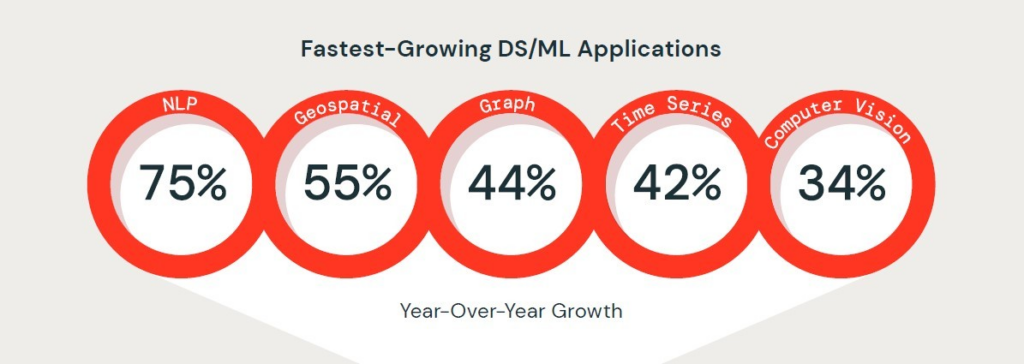
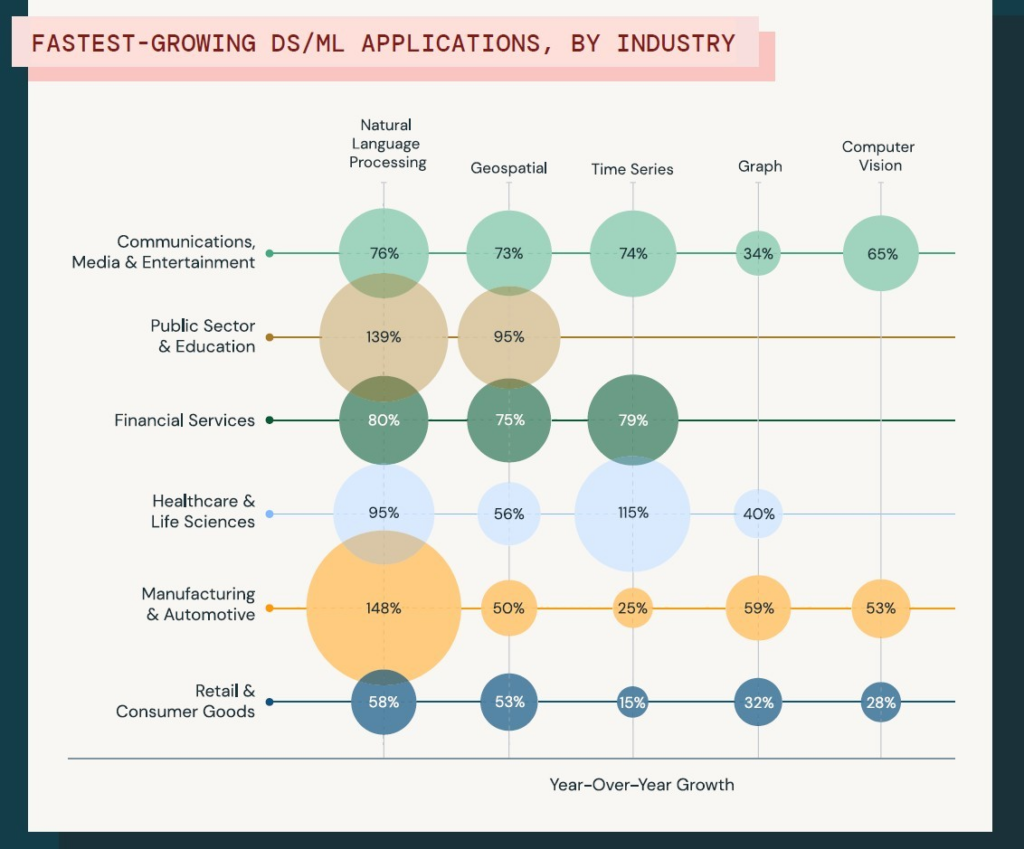
4. The Surge in LLM Customization via Retrieval Augmented Generation (RAG) and Vector Databases: Companies are increasingly focused on customizing LLMs with their private data using the RAG technique to improve accuracy and reduce hallucinations. RAG offers advantages over fine-tuning or pretraining by enabling the incorporation of proprietary, real-time data into LLMs more efficiently. The report notes that, as a result, vector databases, which are essential for RAG, grew 377% YoY, and 70% of companies leveraging GenAI are using tools and vector databases to augment base models.
5. Preference for Open Source AI Tools and Models: In the data and AI product landscape, 76% of companies using LLMs choose open source, often alongside proprietary models, and 9 out of 10 of Databricks‘ top products are open source, according to the report. This indicates a desire for flexibility and avoiding vendor lock-in. Among open-source LLMs, there’s a tendency to select smaller models (with 13B parameters or fewer) likely due to cost and latency considerations.
6. Highly Regulated Industries as Early Adopters of GenAI: Contrary to common perception, highly regulated industries like Financial Services and Healthcare & Life Sciences are emerging as significant early adopters of GenAI. These industries are leveraging open-source LLMs to maintain control over their data while benefiting from GenAI capabilities for industry-specific needs. Financial Services leads in GPU usage, which is crucial for LLM training and serving, showing an 88% growth over 6 months.
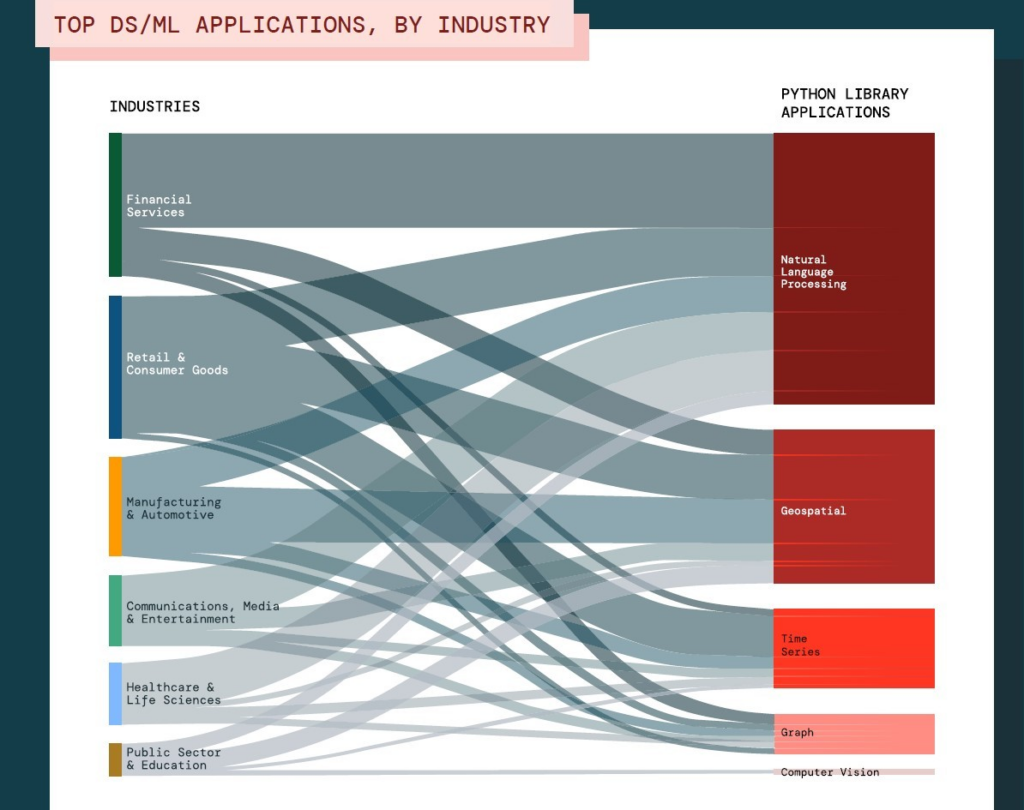
7. Importance of Unified Data and AI Governance: The report highlights the critical role of AI security and governance in building trust in AI initiatives, with Financial Services at the forefront. This reflects the ingrained culture of regulatory and security compliance in these organizations.
8. Shift Towards Serverless Architectures for Real-time ML Applications: Companies are increasingly adopting serverless technologies for data warehousing, monitoring, and model serving to build scalable real-time ML applications. Financial Services (+131% over 6 months) and Healthcare & Life Sciences (+132% over 6 months) are leading in the adoption of serverless products, demonstrating the need for flexible and cost-effective infrastructure to handle fluctuating data processing demands and real-time predictions.
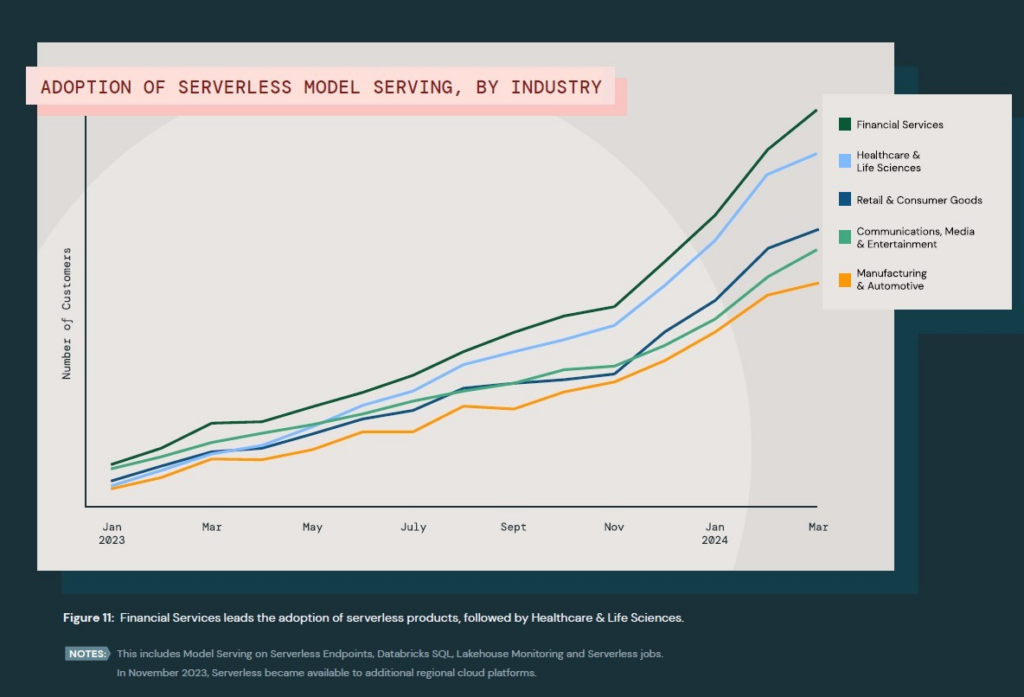
Conclusion
- Across industries, companies are making a decisive shift from experimentation to deployment to production in AI, particularly GenAI – a shift that’s heavily reliant on effectively leveraging enterprise data, leading to the rise of RAG techniques and the importance of vector databases.
- Companies are signaling their demand for greater control and flexibility with their move toward adopting open-source tools and models.
- Highly regulated industries are the fastest to adopt GenAI with robust governance frameworks.
- The future leaders across all industries will be determined by which organizations can most quickly and effectively integrate data and AI.