A new report out from QuantumBlack, AI by McKinsey, a division of McKinsey & Company, offers an operating model for successfully scaling generative AI in an enterprise through a series of data-centric processes.
Today, the OCOLO team will share excerpts from the report that summarize their approach to “moving past the honeymoon phase to embrace the work that matters most: creating value from this tantalizing technology.”
The stakes are high: according to a May 2024 QuantumBlack, AI by McKinsey global survey, 65 percent of companies across sizes, geographies, and industries now use gen AI regularly, twice as many as last year. It is widely accepted that investment in gen AI generates higher performance, lower costs and greater efficiencies over the long term.
With its approach, QuantumBlack, AI by McKinsey seeks to avoid two common traps: 1) tech for tech – allocating significant resources to gen AI without a clear business purpose, leading to solutions disconnected from real-world impact; and 2) trial and error – experimenting with disparate gen AI projects in an uncoordinated manner, lengthening the time it takes to affect productivity.
Here are the steps QuantumBlack, AI by McKinsey proposes for designing an effective gen AI operating model:
Step 1: Design a gen AI operating model around components
This approach allows for adding new gen AI components to the enterprise architecture at regular intervals at ways that are aligned with business goals, and without having to overhaul the tech stack. This way, organizations can be flexible, first implementing the minimum necessary components for critical gen AI use cases, and then adding and removing components as needs evolve.
There are two critical success elements for the plan: 1) a clear road map and 2) a task force to review, update and evolve it during execution. The task force also assigns execution plans, ensuring IT, data, AI, and business teams have appropriate responsibilities for specific rollouts.
Interestingly, the May 2024 study found that even though a component-based approach to gen AI deployment is a crucial success factor for scaling gen AI, only 31 percent of gen AI high-performers and 11 percent of other companies have adopted this model.
Step 2: Choose an extended or distinct gen AI team
Defining a core team is critical to building a gen AI operating team and there are two main options: 1) extend an existing data or IT team by equipping them with new gen AI skills or 2) build a distinct and separate gen AI team.
Making an existing data team responsible for gen AI may seem to be the easier option, though the pendulum could shift as gen AI matures. This approach can limit scope and slow down future rollouts because gen AI products are integrated into the company’s overall technology platform.
Decoupling the gen AI team from the IT or data organization allows an organization to build a new highly skilled gen AI team from scratch with a solid foundation in data and AI architectures that can quickly iterate on gen AI components outside of the larger IT function.
Either model can be successful, but both have pitfalls companies should be careful to avoid. If the gen AI team is decoupled from IT, its road map should still be aligned with the broader IT organization to avoid duplicating efforts or building disconnected gen AI components in multiple places. However, if the gen AI team expands as an offshoot of existing IT and data functions, the team will need to successfully manage two starkly different technology life cycles.
Whether a company chooses an extended or distinct gen AI team, it is important for a central IT team to define a common underlying technology infrastructure that ties all gen AI tools together.
Step 3: Prioritize data management in strategic business domains
Without a functional data organization, gen AI applications will not be able to retrieve and process the right information they need. That is why a data management and governance strategy should be part of any operating model for gen AI. Governance includes managing document sourcing, preparation, curation and tagging, as well as ensuring data quality and compliance, for both structured and unstructured data.
It’s a daunting task to manage the vast amounts of unstructured data that comprise 80% of companies’ overall data. In fact, the May 2024 study cites 60% of gen AI high performers and 80% of other companies struggle to define a comprehensive strategy for organizing their unstructured data. To address this challenge, organizations can prioritize specific domains and subdomains of unstructured data based on business priorities. The ideal domains and subdomains should be small enough to be actionable while being sufficiently large enough to provide a significant, measurable outcome.
The process should be launched by specifically trained data and natural-language-processing engineers, grouped into a CoE, who establish and implement processes for managing unstructured data, provide a view on when and where data is consumed, and ensure consistent standards for data quality, risk management, and compliance.
One important point: once the CoE provides a deployment road map, domain experts with business oversight should take over the data management process, because they are better equipped to extract knowledge from specific records in their field than data professionals alone.
Step 4: Plan for a decentralized approach to gen AI development
As domain teams become more adept at managing data, forward-thinking data executives may want to ensure their gen AI operating road maps include future scenarios of decentralized development.
There are three approaches to consider: 1) Centralized gen AI – centralizing gen AI on a company’s own domain allows an organization to build capabilities quickly and control costs. This approach keeps development costs low and reduces the risk of multiple teams creating similar projects. 2) Federated gen AI – as companies build gen AI expertise, they often choose a federated model, where business units are not only responsible for consuming data related to their domains but also take over data processing and repositories. This model allows domains to integrate gen AI more deeply into their daily workflows for stronger business outcomes. 3) Decentralized gen AI – in this model, each domain creates its own gen AI team composed of business, data, and technical experts aligned on a common goal to develop relevant gen AI applications. A decentralized model of gen AI development allows domains to create gen AI agents tailored specifically to their needs, which they can then offer to other domains. With this approach, it is important for a centralized IT team to retain visibility into the tools being developed to avoid blind spots and ensure no two teams develop similar gen AI tools.
This is what the 3 frameworks look like side-by-side:
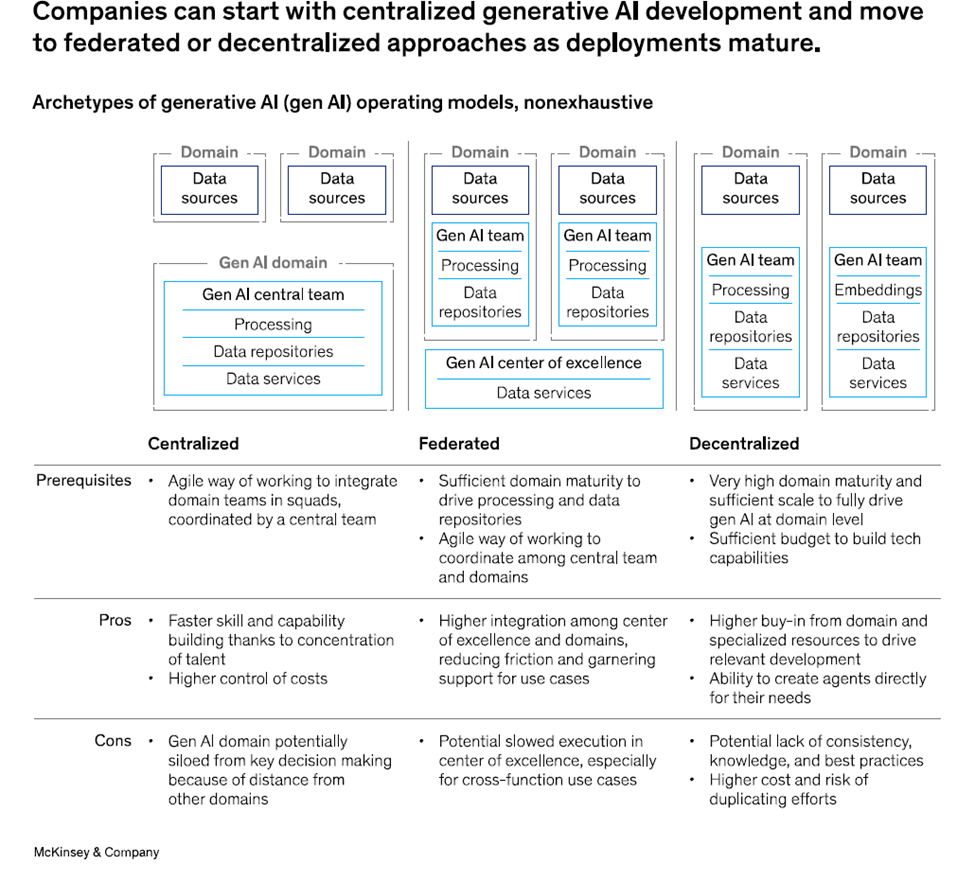
Step 5: Unify federated teams through common infrastructure
A decentralized development process should never compromise the company’s overall security or resiliency. Companies must ensure IT teams build and manage an underlying common infrastructure on top of which all gen AI tools are developed and deployed. The IT team should also be responsible for building repeatable platforms that can be used by all the business units, such as a prompt library, a repository for Python code, standard agents, and systemized cloud storage. This type of centralized IT management empowers business units to create new gen AI tools, while ensuring all use cases they develop adhere to a highly secure and unified technology framework.
Step 6: Emphasize risk and compliance governance
Gen AI comes with heightened risks, including potential hallucinations, misinformation, and data leaks, so every gen AI operating model should include explicit stipulations for risk and compliance governance. Creating a gen AI risk plan involves a six-step process that data leaders must continually monitor and update as new potential risks arise and when new tools are deployed:
- Identify new risks: Ask developers and technology users to identify potential AI-specific threats to add to the company’s overall risk plan.
- Classify gen AI tools: Encourage data teams to collaborate with the risk function to apply oversight to the most critical gen AI tools first.
- Deploy a tiered approach: Adjust the depth and frequency of derisking methods for each gen AI tool based on continual risk assessments.
- Make risk tracking habitual: Begin oversight at the development stage, continuing to measure risks throughout implementation and production.
- Equip risk teams for success: Establish a CoE with developers and risk leaders to ensure the risk team keeps pace with evolving gen AI trends.
- Get everyone on board: Ensure end users, developers, managers and leaders all understand the company’s policies for safe gen AI use.
Clearly, the team at QuantumBlack, AI by McKinsey and McKinsey & Company coordinated extensively on a global level to deliver this comprehensive blueprint for companies to scale their gen AI operating models for long-term success. We’d like to thank the study’s authors cited as well as their cited contributors:
Alex Singla is a senior partner in McKinsey & Company’s Chicago office; Dr. Asin Tavakoli is a partner in the Düsseldorf office, where Holger Harreis is a senior partner; Kayvaun Rowshankish is a senior partner in the New York office; Klemens Hjartar is a senior partner in the Copenhagen office; and Gaspard Fouilland and Olivier Fournier are consultants in the Paris office.
The authors wish to thank Jean-Baptiste DUBOIS, Jon Boorstein and Pedro J Silva for their contributions to this article.