Last week’s DCD>Academy Connect NYC Conference covered a lot of ground across its three tracks – Compute, Connect, Investment – underscoring the theme of rapid growth and evolution in all aspects of digital infrastructure. Nowhere is this more evident than in Artificial Intelligence (AI), which, before our very eyes, has already transitioned from AI to Generative AI (GenAI), with use cases maturing from testing applications (training) to implementing (working) to, ultimately, distributing (inference).
This seems straightforward, but like everything with AI, there’s actually a lot to unpack. Each stage of AI’s evolution requires more capital and more compute power to execute, so looking down the road at what future use case eras of GenAI might look like produces some mind-boggling numbers in terms of both dollars and units of energy.
In its March Telecom & Digital Infrastructure Market Update, Guggenheim Securities‘ Telecom & Digital Infrastructure Investment Banking Team chose the evolution of GenAI infrastructure from the model training phase to inference as their “Topic of Month.” The authors use data from Barclays Research’s brilliant “ImpactSeries” AI report published in January and other sources to produce a visual of how this evolution is playing out between 2023-2028. It’s both fascinating and scary stuff, as you can see below.
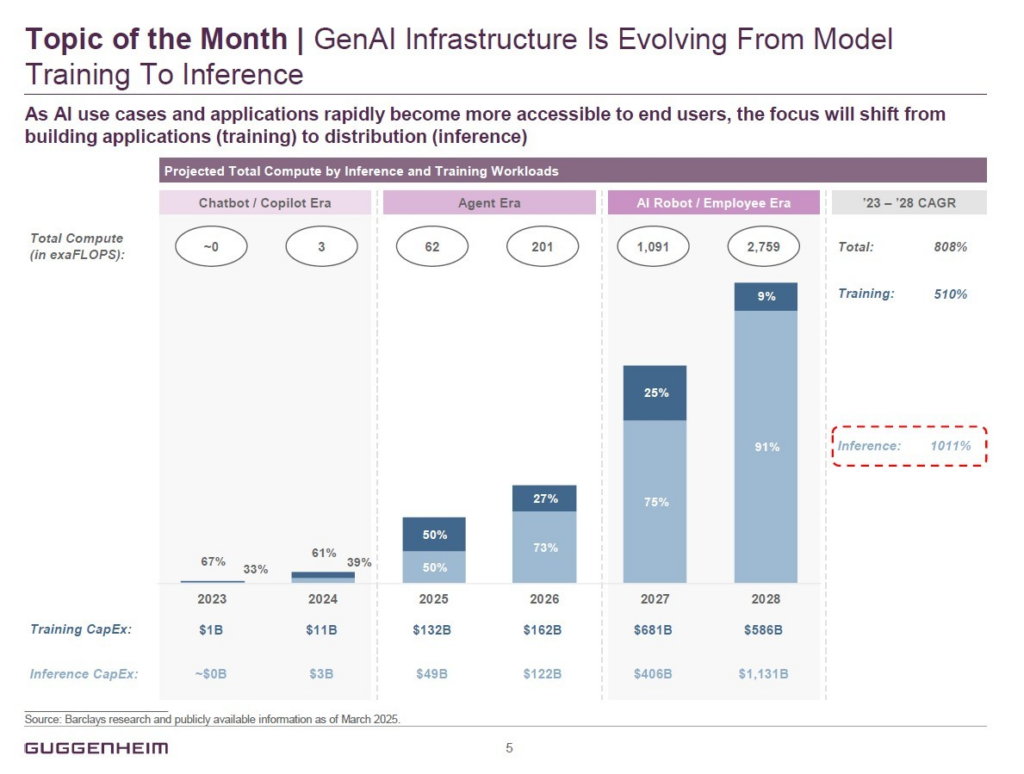
Key Takeaways
3 Eras in 8 Years
Did you know we’re already past the first Era? According to the Guggenheim Team’s thesis, we are in the beginning of Era 2 of a 3-Era cycle that’s happening between 2023-28. The Chatbot / Copilot Era from 2023-24 was the first, and it could just as easily be called “The AI to Generative AI Era,” because it’s when all the models were built, first to address basic processes like pattern recognition or task automation, then on to analytics and prediction, and then ultimately to Generative AI models that actually create context like text, images, code, music, video, 3D models and more. The capex in those early days was primarily in training and ramped in an astonishing fashion: from $1 billion in 2023 to $11 billion in 2024. Already, $3 billion had poured into inference capex by end-2024 – another sign of how swiftly programmers were making progress.
The Heat is On
The visual suggests that our current Era, the Agent Era, is the one where spending on Training and Inference will balance out before Inference spend pulls permanently and decisively into the lead – from 50/50 for 2025 to closer to 75/25 in favor of Inference for 2026. What’s even more astounding is the increase in spend that’s driving the size of the two bars. From $11 billion in 2025, training capex is expected to leap to $132 billion in total for 2025 and another $162 billion for 2026! And Inference capex, which was just $3 billion in 2024, is projected to hit $49 billion in 2025, then more than double from that to $122 billion in 20226!
Where is all this spend going? It’s all in the name: The Agent Era is named for Agentic AI, the next-next-generation of AI already under development that is designed to exhibit goal-directed behavior, act autonomously in pursuit of objectives, make decisions and learn from its environment. In other words, Agentic AI is AI that behaves like an intelligent agent, capable of initiating actions based on its own assessments, following through and redirecting its approach based on findings along the way. Not scary at all, right?
Now’s the time in the program when we address the compute power that creating this kind of complex and sophisticated technology requires, shown in the visual “in exaFLOPs”. According to the Barclays ImpactSeries AI report that the Guggenheim team used for this visual, at the current rate of scaling, AI models could start to exceed a billion exaFLOPs by 2026 and hundreds of billions of exaFLOPs by 2028. For the uninitiated: one exaFLOP = a billion, billion floating point calculations per second. Put another way, a quintillion (10 to the 18th power), or 1,000,000,000,000,000,000. It is a measure of computational speed used to quantify the performance of high-powered processors or systems, especially in AI and supercomputing.
Training a single model could require several, if not tens of, gigawatts of power. For instance, training GPT-4 reportedly consumed several hundred thousand GPU hours, adding up to tens of exaFLOPs over time. Look at the bubbles across the top of the visual and see how compute power ramps substantially in the Agent Era. Now look at total compute for the AI Robot / Employee Era that’s supposedly just two years away. Mind blown.
New Math
There’s always a point in telling growth stories about AI where the numbers just look silly and we’re well past it with this one. There are a lot of good candidates, like exaFLOPs, as well as the numbers for both total capex and capex CAGR, on the visual above. Using the information available, the team estimates that Training capex CAGR will be 510% between ’23-’28 and Inference capex will be 1,011%, for a total of 808%. Inference capex is expected to top $1.131 trillion in 2028, about double the spend on Training capex that same year and more than triple Inference spend in 2027. In fact, the only number that is actually lower year-on-year in the entire visual is Training capex in 2028, and we can only believe that’s because the shift in AI use cases from applications (training) to distribution (inference) will be nearly complete by then.
What a difference 6 years makes! What do you think? Can you fathom this much growth in just 6 years? Is the AI Robot / Employee Era really just under two years away? Will AI get that scary smart and self-directed that quickly? We love comments!